Risk management for generative AI apps
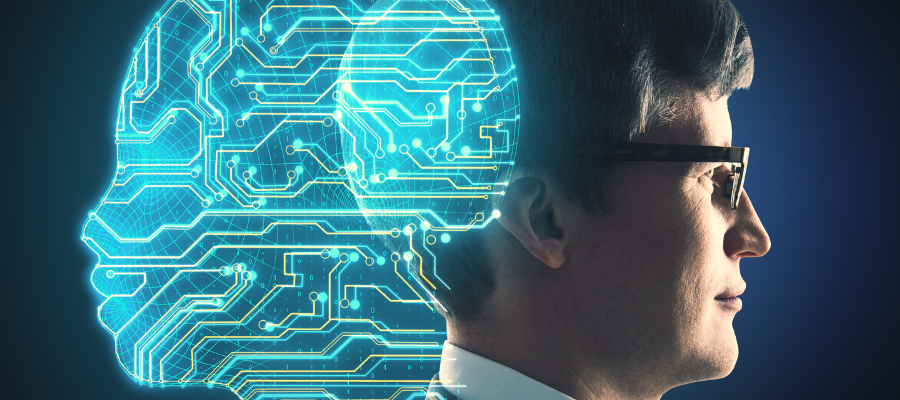
Generative AI refers to artificial intelligence systems that are capable of creating new and original outputs, such as images, music, and text. Unlike traditional AI systems that are trained to recognize patterns and make predictions based on existing data, generative AI systems are designed to generate new outputs based on a learned model of the data.
Generative AI is revolutionizing how products are developed and quickly proving how creative work can be derived automatically from a machine-learning algorithm. This comes with substantial impacts on automated decision-making, marketing, software, and content creation.
However, Generative AI systems will likely face intense scrutiny in the future.
Risks of Generative AI
US Congressmen Don Beyer and Rep. Ted Lieu suggested that a new agency should be created to regulate A.I., similar to the FDA, staffed with experts and able to reverse AI decisions if needed. At an event in Stockholm, Nvidia tools were announced to upgrade Sweden's fastest supercomputer to develop a large language model fluent in Swedish, though Huang, Nvidia CEO warned of potential harm.
Generative AI apps need to consider several risks, including:
Bias and fairness: Generative AI models can perpetuate and amplify existing biases in their training data, leading to unequal and unfair outcomes for certain groups.
Misuse and abuse: Generated content can be used for malicious purposes, such as creating fake news or deepfakes.
Legal and ethical implications: Generated content can raise questions about ownership, intellectual property, and privacy rights.
Technical limitations: Generative AI models can generate low-quality or nonsensical outputs, leading to confusion and miscommunication.
To mitigate these risks, developers of generative AI apps need to perform regular bias audits, implement robust moderation and filtering systems, engage in the transparent and responsible use of AI, and continuously monitor and improve the performance of their models.
Failing to plan such audits, teams might be putting themselves at unknown risks like:
- Legal and regulatory consequences: AI models that are found to be discriminatory or biased can result in legal action and regulatory fines.
- Reputational damage: Models that perpetuate biases and discrimination can damage the reputation of the organization and erode public trust.
- Ineffective and inefficient models: Models that are biased can produce poor results and generate inaccurate outputs, leading to ineffective and inefficient decisions.
In 2020, The European Commission's proposal for the regulation of AI includes fines of up to 6% of a company's global annual turnover.
There are plenty of cases where organizations were fined: UK-based bank fined $85 million for AML process failures and Clearview AI was fined $9.4 million, among the most notable.
Conducting Internal Audits
Regulators are increasingly taking notice of AI incidents, and standards and frameworks are becoming more concrete, so audits and other risk management controls should be expected in the future of AI audit and risk management.
Neglecting to conduct internal bias audits can have serious consequences for both the organization and its stakeholders. It is therefore critical for AI teams to prioritize the regular evaluation and mitigation of bias in their models.
To conduct various audits (bias, fairness, transparency) internally with your whole team, you can follow these steps:
Define the scope of the audit: Determine the specific aspects of the model's behavior and outputs that you want to evaluate for bias.
Gather and analyze data: Collect data on the model's inputs and outputs and analyze it for patterns of bias. This could involve calculating demographic disparities in outcomes, examining the model's decisions for fairness, or examining the representation of different groups in the training data.
Consult with subject matter experts: Seek out experts in relevant fields such as ethics, fairness, and diversity, and engage in open discussions on potential biases and their implications.
Engage in ongoing monitoring and improvement: Regularly evaluate the model's performance and make necessary updates to address identified biases. This could involve adjusting the model's architecture, fine-tuning its parameters, or collecting and incorporating additional diverse training data.
Communicate findings and remediation steps: Clearly communicate the results of the audit, any biases identified, and the steps being taken to address them to stakeholders, including customers, employees, and regulators.
It's important to note that bias audits are an ongoing process, and it's crucial to regularly evaluate and update the model to ensure it remains unbiased and fair.
BNH.AI, a boutique law firm focused on AI risks, audited FakeFinder, a deepfake detector, and an LLM for NER tasks, and found that bias in AI can arise from a variety of sources, such as decisions in initial meetings, homogenous engineering perspectives, improper design choices, and insufficient stakeholder engagement, and that organizations should start with simple metrics and convert AI outcomes to binary or numeric outcomes to avoid confusion and delay.
Test Collab helps AI teams plan such internal audits collaboratively so that work is evenly distributed among your QA and developers. It also helps quantify the outcomes of such testing, so your team can make clear decisions
Audit Logs for AI application testing
Although we’re still in the early phases of AI apps, we recommend dev teams keep audit logs from the early phases of the project. While this increases a little bit of effort upfront, the benefits greatly outweigh the risks. There are several benefits once you automate this:
Monitoring progress: Old audit records provide a historical record of the model's behavior and performance, allowing AI teams to track their progress and evaluate the effectiveness of any remediation steps taken.
Legal and regulatory compliance: In some cases, organizations are required to maintain audit records for a certain period of time to meet legal and regulatory requirements.
Auditability: Old audit records provide a clear and detailed history of the model's development and evolution, making it easier to audit the model's behavior and ensure its compliance with ethical and legal standards.
Improving future models: Retaining old audit records can inform the development of future models by providing insight into past issues and their resolution, helping to avoid repeating mistakes and improve future models.
In conclusion, keeping old audit records is crucial for ensuring the continued development, evaluation, and improvement of AI models, as well as for meeting legal and regulatory requirements and establishing a clear and transparent audit trail.
Test Collab offers its users the ability to create collaborative Test Plans which can be used for record-keeping and tracking improvements of AI models over time.
In the end, we also recommend QA professionals and development teams working with AI apps be aware of AI ethics proposed by interdisciplinary organizations, such as Montreal AI Ethics Institute or other AI NGOs.